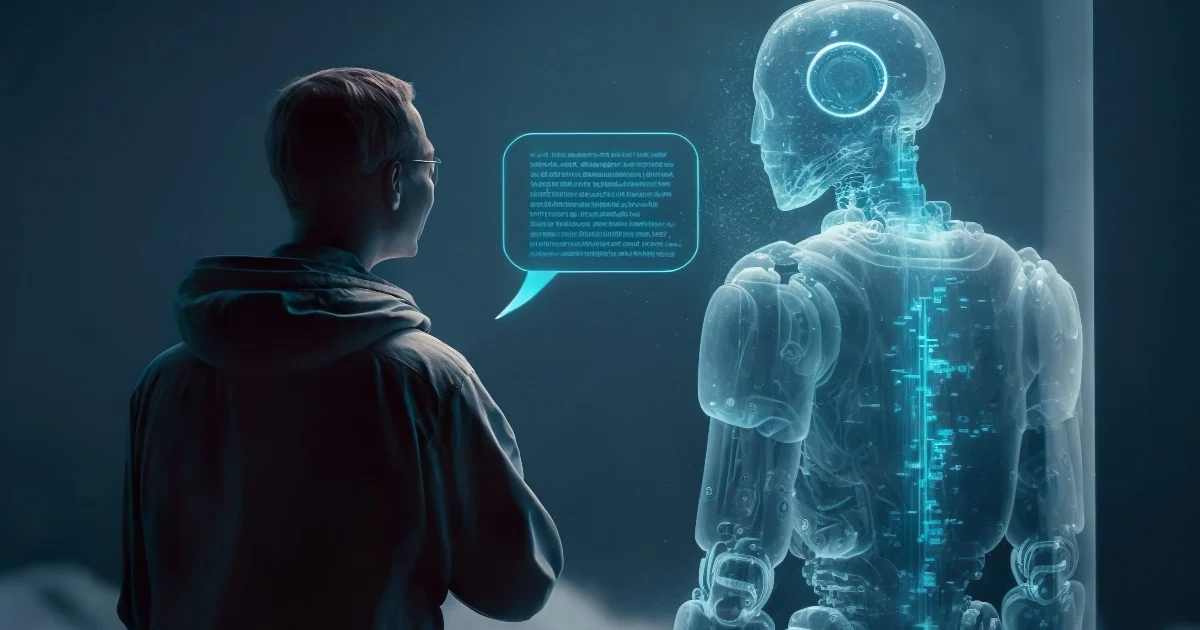
AI agents are sophisticated software programs designed to perform tasks autonomously, leveraging artificial intelligence to enhance efficiency and productivity across various business applications. This article explores the definition of AI agents, their functionalities, types, real-world examples, comparisons with traditional software solutions, and their impact on business efficiency and productivity.
Definition and Functionalities
AI agents are systems that can perceive their environment, reason about it, and take actions to achieve specific goals. They operate through a combination of data collection, learning, and decision-making processes. The core functionalities of AI agents include:
- Goal Setting: AI agents can set and pursue specific objectives, whether defined by users or autonomously determined.
- Data Collection and Understanding: They gather data from various sources, including user interactions, to inform their decisions.
- Decision Making: Using algorithms and machine learning, AI agents analyze data to make informed decisions and predictions.
- Learning and Adaptation: AI agents improve their performance over time by learning from past interactions and outcomes.
Types of AI Agents in Business Applications
AI agents can be categorized into several types, each suited for different business needs:
Simple Reflex Agents
At the foundation level, simple reflex agents operate on straightforward “if-this-then-that” decision-making principles. They perceive their environment and react based on predefined rules without maintaining any internal state. A smart thermostat exemplifies this category perfectly—when it detects the room temperature has fallen below a set threshold, it automatically activates the heating system to restore comfort levels in your shop.
- Example: A smart thermostat that adjusts temperature based on current conditions.
Model-Based Agents
Moving up in complexity, model-based agents maintain internal representations of their surroundings. These agents don’t merely respond to immediate stimuli but create comprehensive models of their environments to track changes and predict outcomes before taking action. Consider a pizza delivery robot as an illustration: it remembers traffic regulations, observes pedestrian behaviors, and constructs mental models from these observations to determine optimal delivery routes that ensure customers receive fresh, hot pizza.
- Example: A pizza delivery robot that navigates based on traffic patterns and pedestrian behavior.
Goal-Based Agents
Goal-based agents represent another advancement in the evolution of AI systems. These sophisticated agents evaluate current conditions alongside multiple potential future scenarios to determine which actions will best achieve specified objectives. When a particular strategy proves ineffective, goal-based agents employ reasoning capabilities to adapt their approach. Digital marketing agencies might implement such agents to assess and prioritize client leads by reviewing website inquiries, scoring prospects based on fit and conversion potential, initiating appropriate communications, and assigning leads according to urgency and quality scores.
Utility-Based Agents
When situations involve multiple competing outcomes, utility-based agents excel by assigning numerical values to each potential result and selecting actions that maximize overall benefit or minimize harm. An inventory management system serves as a prime example of utility-based AI in action. Such a system balances competing priorities such as preventing stock shortages, maintaining healthy inventory turnover, and minimizing procurement costs. By analyzing sales patterns and monitoring vendor pricing fluctuations, these systems purchase products at optimal times and quantities to achieve balanced outcomes across all objectives.
- Example: An inventory management system that balances stock levels, turnover rates, and costs.
Learning-Based AI Agents
The most advanced category encompasses learning-based agents, which continuously improve performance through accumulated experience. These systems modify their decision-making strategies and develop increasingly sophisticated problem-solving approaches over time. Netflix’s recommendation engine perfectly demonstrates this capability—it tracks viewing habits and learns individual preferences to progressively refine its content suggestions, creating an increasingly personalized experience with each interaction.
- Example: Netflix’s recommendation engine that refines suggestions based on user viewing habits.
Examples in Real-World Scenarios
AI agents are already making significant impacts across various industries. Here are some notable examples:
- Customer Support: AI chatbots like Forethought provide context-aware responses to customer inquiries, improving service efficiency and satisfaction.
- Lead Management: LocaliQ’s Dash AI agent helps businesses manage incoming leads by summarizing calls, categorizing leads, and prioritizing follow-ups.
- Conversational E-commerce: Bloomreach offers an AI agent that assists shoppers in finding products through personalized interactions across multiple channels.
- Social Media Management: Sprout Social employs AI agents to automate post scheduling, generate replies, and analyze brand sentiment.
- Human Resources: HiredScore AI by Workday aids recruiters by screening candidates and providing insights to prioritize hiring.
AI Agents vs. Traditional Software Solutions
AI agents differ significantly from traditional software solutions in several ways:
Feature | AI Agents | Traditional Software Solutions |
---|---|---|
Learning Capability | Continuously learn and adapt from interactions | Static; requires manual updates and changes |
Decision Making | Autonomous decision-making based on data | Predefined rules and logic |
Data Processing | Handles large volumes of data in real-time | Limited data processing capabilities |
User Interaction | Engages users in a conversational manner | Typically relies on forms and static inputs |
Operational Hours | 24/7 availability | Limited to business hours |
Impact on Business Efficiency and Productivity
The integration of AI agents into business operations can lead to significant improvements in efficiency and productivity:
- Time Savings: AI agents automate repetitive tasks, allowing employees to focus on strategic initiatives. For instance, an AI agent can manage lead categorization and follow-ups, freeing up hours for sales teams to engage with high-priority prospects.
- Improved Accuracy: By minimizing human error, AI agents ensure consistent and accurate outputs. This is particularly beneficial in customer service, where AI can provide reliable answers to inquiries.
- Enhanced Customer Experience: AI agents can provide instant responses and personalized interactions, improving customer satisfaction and loyalty. For example, Bloomreach’s conversational shopping agent enhances the shopping experience by guiding users through their purchasing journey.
- Data-Driven Insights: Analyze vast amounts of data to provide actionable insights, helping businesses make informed decisions. This capability is crucial in marketing, where understanding customer behavior can lead to more effective campaigns.
AI Agents in Lead Generation and Management
AI agents play a pivotal role in lead generation and management by automating processes that traditionally required significant human effort. Key functionalities include:
- Lead Scoring: Evaluate leads based on predefined criteria, helping sales teams prioritize their efforts.
- Automated Follow-Ups: They can send personalized follow-up messages to leads, ensuring timely communication.
- Data Analysis: Analyze lead interactions to identify patterns and optimize future outreach strategies.
For example, LocaliQ’s Dash AI agent streamlines lead management by categorizing leads and providing summaries of interactions, enabling businesses to respond more effectively.
Future Trends in AI Agents and Automation
The future of AI agents is promising, with several trends expected to shape their development and application:
- Increased Personalization: They will leverage advanced data analytics to provide even more personalized experiences for users, enhancing customer engagement.
- Integration with IoT: As the Internet of Things (IoT) expands, AI agents will increasingly interact with connected devices, enabling smarter automation in various sectors.
- Enhanced Natural Language Processing (NLP): Improvements in NLP will allow AI agents to understand and respond to human language more effectively, making interactions more seamless.
- Ethical AI Development: As AI becomes more prevalent, there will be a greater focus on ethical considerations, including transparency, accountability, and bias mitigation in AI systems.
- Collaboration with Human Workers: AI agents will complement human efforts rather than replace them, leading to hybrid work environments where AI handles routine tasks while humans focus on complex decision-making.
Conclusion
AI agents are a transformative force in the business landscape, offering capabilities that enhance efficiency, accuracy, and customer satisfaction. By understanding the types, functionalities, and real-world applications of AI agents, businesses can leverage these tools to gain a competitive advantage and drive growth. As technology continues to evolve, the potential to reshape industries and improve operational processes will only increase, making them an essential component of modern business strategy.